Machine learning is a powerful tool that can help organizations unlock new insights and drive better business outcomes. However, as with any new technology, there is a learning curve. This blog post will explore some of the worst mistakes you can make when implementing machine learning. By avoiding these pitfalls, you can set your organization up for success.
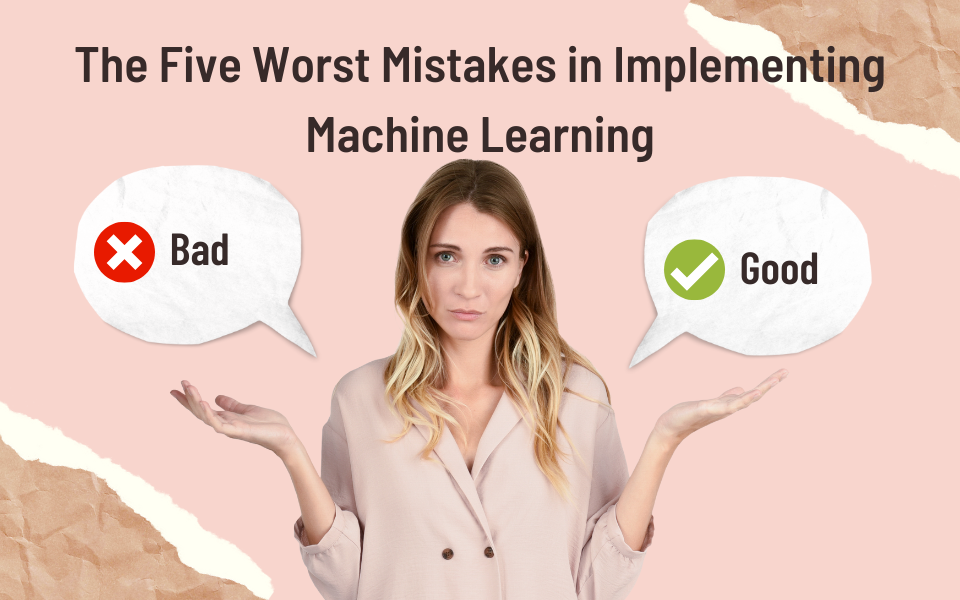
1. Not Defining the Objective Early On
One of the most common mistakes in implementing machine learning is failing to define the objective early on. Without a clear and concise objective, it can be difficult to determine which data is relevant and which algorithms will be most effective. This can lead to suboptimal results and wasted time and resources.
2. Not Cleaning the Data
Another mistake that is often made is not cleaning the data sufficiently before training the machine learning model. Dirty data can lead to inaccurate results and suboptimal performance. It is important to clean the data thoroughly so that the machine learning model can be trained on high-quality data.
3. Training on Too Much Data
A common mistake that is made is training on too much data. While it may seem counterintuitive, too much data can actually lead to overfitting, which means that the machine learning model does not generalize well to new data. It is important to strike a balance between training on enough data so that the model can learn effectively but not so much that it overfits.
4. Not Monitoring Model Performance
It is also important to monitor model performance after training and during deployment. This allows you to catch any issues early on and prevent them from later causing major problems. Often, issues can arise after a deployed model that was not apparent during training due to changed conditions or different types of data being used.
5. Not Updating the Model Regularly
Another mistake that is often made is failing to update the machine learning model regularly. As new data becomes available, it is important to retrain the model to stay current and perform well. Additionally, as conditions change, it may be necessary to adjust the model so that it continues to meet the objective defined at the beginning of the project.
Conclusion:
Machine learning is a powerful tool that can help organizations derive insights and drive better business outcomes—but only if it’s used correctly! This blog post explored some of the worst mistakes you can make when implementing machine learning projects. By avoiding these common pitfalls, you can set your organization up for success.
Thanks for reading! We hope this blog post helped steer you away from common machine learning implementation mistakes.