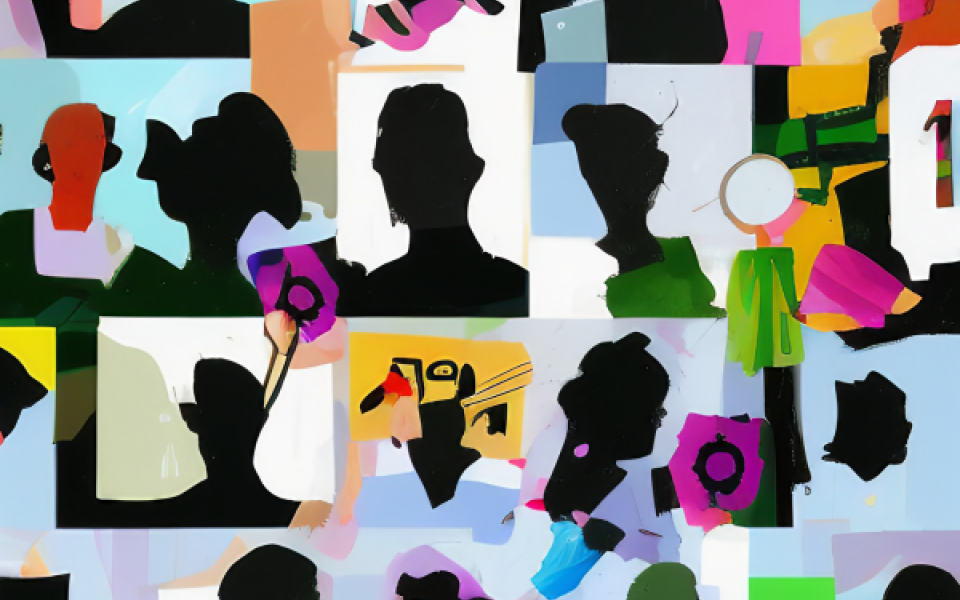
It’s important to stay up-to-date with the latest trends in MLOps and AutoML. As these two fields are converging, key trends are emerging. In recent weeks, I’ve spoken with over 20 ML practitioners to gain insight into their vision for the future of MLOps. Here’s what we can expect in the years ahead:
1. Closing the loop in machine learning systems. Companies are moving beyond ML pipelines that adhere to unidirectional flows from data to predictions.
2. Declarative systems for machine learning. We’re beginning to see a trend towards “Webflow for ML”, which allows for easier and more dynamic system development.
3. Real-time machine learning. Organizations are investing in real-time ML infrastructure solutions to ensure fresher models with fresh data.
4. Better data management. As “data is the new electricity”, companies need better tools to manage data across its lifecycle, from sourcing and curating to labelling and analyzing.
5. Merging business insight tooling with data science/machine learning workflows. As ML outputs drive more value for organizations, there is a need to align the BI and ML stacks.
6. Talent shortage remains rampant. The demand for quality ML talent is outpacing the current supply, making MLOps tooling an attractive investment.
7. Cultural adoption of machine learning thinking. Organizations must adopt an ML mindset and embrace continual experimentation to realize its full potential.
8. Increasing consolidation around end-to-end platforms. E2E solutions are becoming more popular as they offer convenience of use.
As these trends shape the MLOps space, companies must understand how to best leverage them to ensure successful implementations and realize their desired outcomes. With a better understanding of where the industry is heading, businesses can develop more effective strategies for leveraging ML and data science to drive their business forward.